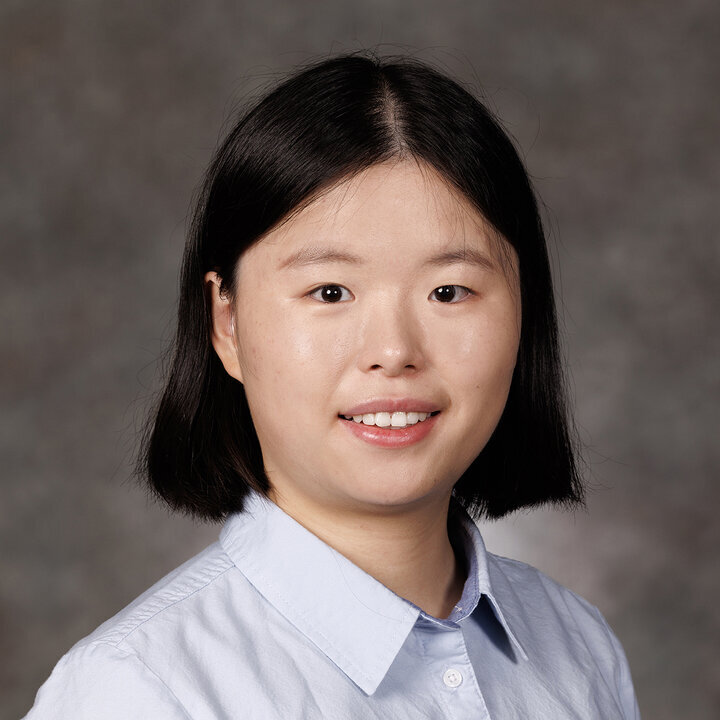
Tianjing Zhao
Asst Professor Animal Science University of Nebraska-Lincoln
Contact
- Address
-
ANSC A218f
Lincoln NE 68583-0908 - Phone
-
-
Dr. Tianjing Zhao joined the Department of Animal Science in August of 2023 and currently serves as an assistant professor in theoretical quantitative genetics.
Education
Ph.D., Statistical and Quantitative Genetics, University of California-Davis, 2023
M.S., Statistics, University of California-Davis, 2019
B.S., Economic Statistics, Southwestern University of Finance and Economics (China), 2017
Teaching
ASCI 865: History and Perspectives in Animal Breeding and Genetics
Summary: Historical perspective to the discipline of animal breeding and genetics. Introduction to the contributions of geneticists who have significantly impacted the discipline. Material includes pre-recorded interviews of scientists that have had an international impact in animal breeding and genetics. Critique key papers.
ASCI 496/896: Independent Study in Animal Science (Statistical Genomics)
Summary: This course will introduce concepts in quantitative genomics to study the genetic architecture of complex traits. We will discuss statistical methods and software tools for analyzing complex traits with high-dimensional genomic data from both predictive and inferential perspectives. Topics will include genomic relatedness, genomic heritability, linear mixed models, mixed model equations, variance component estimation, Bayesian Alphabet, genomic prediction, GWAS, multi-trait models, summary statistics-based models, incorporating multi-omics into mixed models, incorporating functional annotation into mixed models, GxE interactions, etc.
Research
The Statistical and Quantitative Genetics Lab (https://tianjing.one/) at the University of Nebraska–Lincoln focuses on addressing challenges in the era of big data and multi-omics data to improve our understanding of the genetic architecture of complex traits through the development of statistical methodologies. Specifically, we: 1) develop new statistical models to incorporate emerging data (e.g., multi-omics) into genomic data analysis, 2) contribute to the development of software tools for genomic data analysis, 3) create computational algorithms for large-scale data analysis, and 4) perform statistical analyses in quantitative genetics.
Selected Publications
- Tianjing Zhao, Fangyi Wang, Richard Mott, Jack Dekkers, and Hao Cheng. Using encrypted genotypes and phenotypes for collaborative genomic analyses to maintain data confidentiality. GENETICS, 2023. https://doi.org/10.1093/genetics/iyad210
- Jinghui Li, Tianjing Zhao, Dailu Guan, Zhangyuan Pan, Zhonghao Bai, Jinyan Teng, Zhe Zhang, Zhili Zheng, Jian Zeng, Huaijun Zhou, Lingzhao Fang, and Hao Cheng. Learning functional conservation between human and pig to decipher evolutionary mechanisms underlying gene expression and complex traits. Cell Genomics, 2023. https://doi.org/10.1016/j.xgen.2023.100390
- Tianjing Zhao, and Hao Cheng. Interpreting single-step genomic evaluations as mixed effects neural networks of three layers: pedigree, genotypes, and phenotypes. Genetics Selection Evolution, 2023. https://doi.org/10.1186/s12711-023-00838-7
- Tianjing Zhao, Jian Zeng, Hao Cheng, Extend mixed models to multilayer neural networks for genomic prediction including intermediate omics data, GENETICS, 2022, https://doi.org/10.1093/genetics/iyac034
- Tianjing Zhao, Rohan Fernando, and Hao Cheng. Interpretable artificial neural networks in-corporating Bayesian alphabet models for genome-wide prediction and association studies, G3 Genes|Genomes|Genetics, 2021, https://doi.org/10.1093/g3journal/jkab228
- Tianjing Zhao, Rohan Fernando, Dorian Garrick, and Hao Cheng. Fast parallelized sampling of Bayesian regression models for whole-genome prediction. Genetics Selection Evolution, 2020. https://doi.org/10.1186/s12711-020-00533-x